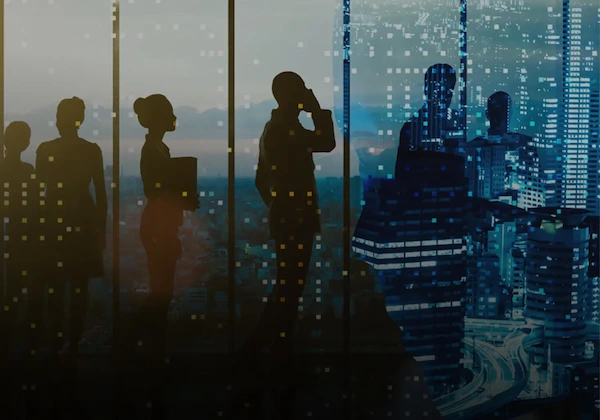
Architects of Singapore’s Digital Future
IMDA leads Singapore's digitalisation journey by developing a vibrant digital economy and an inclusive digital society.
Show profile guideBe digitally empowered for innovation and growth
At IMDA, we empower businesses to leverage opportunities brought forth by digitalisation, through programmes that will aid them in integrating tech, while strengthening their digital foundations.
Get access to tech and media opportunities and upskilling
At IMDA, we endeavour for every Singaporean to be tech-skilled to leverage jobs and opportunities as part of our efforts to build a Digital Talent hub that is globally competitive.
Embrace digital for life. Be enriched by digitalisation, securely
At IMDA, we ensure a safe and inclusive digital society, where all Singaporeans can participate in Singapore’s digital future, and be enriched as we embrace digital for life.